Research Mission
Our lab research is directed towards an overarching goal of developing and validating quantitative magnetic resonance imaging and spectroscopy techniques for various translational and clinical applications. Our research is primarily divided into two areas – a) developing confounder-free magnetic resonance imaging (MRI) methods for accurate quantification of iron overload and steatosis (fatty liver) non-invasively for replacing tissue biopsy, and b) developing automated techniques for quantifying metabolites in biological tissue samples using nuclear magnetic resonance (NMR) spectroscopy.
Current Projects
Quantitative MRI
Assessment of Hepatic Iron Overload and Steatosis
Abnormal accumulation of iron (iron overload), fat (steatosis) and collagen (fibrosis) are common manifestations of diffuse liver diseases which can progress through progressive fibrosis, irreversible cirrhosis and ultimately liver failure if timely interventions are not provided. MRI has emerged as a clinically important non-invasive quantitative tool for assessing hepatic iron overload, steatosis and fibrosis, independently. However, these pathologies often co-exist to varying degrees and will have interfering effects on the acquired MRI signal and can thus bias the assessments of iron overload and steatosis. Our lab research focuses on understanding the interfering effects of these co-existing pathologies on MRI signal by constructing computational tissue models and complex anthropomorphic phantoms and developing techniques that will enable confounder-free assessments of iron overload and steatosis for clinical applications.
Morphological Modeling and Monte Carlo Simulations
Our lab is investigating MRI-based transverse tissue relaxivity (R2*) in presence of iron overload and steatosis to determine an accurate MRI signal model for simultaneous assessment of iron content and fat fraction (FF). We are developing virtual hepatic iron overload and steatosis models based on tissue histology using morphological and statistical descriptors and generating MRI signal using Monte Carlo simulations to predict the tissue relaxivity in iron overload, steatosis and combined iron and fat overload conditions. This research will aid our understanding and quantification of iron and fat mediated relaxivity in tissues and therefore, will help us to develop and validate accurate signal models that can simultaneously quantify R2* and FF, thus enabling the noninvasive diagnosis of both iron overload and steatosis with MRI and replacing tissue biopsy. The web application for hepatic steatosis simulation can be accessed at: https://simhepaticsteatosis.pythonanywhere.com/
Hepatic Steatosis Modeling and MRI Signal Synthesis
Phantom Studies
Phantoms are used as stand-ins for human tissue in both normal and pathological conditions and help facilitate prompt and accurate testing and optimization of MRI methods without the need for living subjects. Our lab constructs realistic iron and fat phantoms for mimicking hepatic iron overload and steatosis conditions to investigate MRI signal models for accurate and simultaneous estimation of R2* and FF, which are predictors for iron overload and steatosis, respectively.
Phantom R2* and Fat Fraction Estimation
Clinical Studies for Iron Overload Assessment
This is a collaborative project with St. Jude Children’s Research Hospital for investigating a multispectral auto regressive moving average (ARMA) and quantitative susceptibility mapping (QSM) to measure magnetic susceptibility as a confounder-free predictor of hepatic iron concentration (HIC). ARMA is a comprehensive technique for simultaneously quantifying R2*, FF, and field maps from a single multi-echo gradient-echo (GRE) acquisition. ARMA R2* maps produce dry HIC estimations, FF maps provide assessment for steatosis and ARMA-generated field maps when used in conjunction with QSM, provide susceptibility-based wet HIC measurements.
Deep Learning for Iron Overload Assessment
Non-invasive assessment of hepatic iron content (HIC) typically involves using biopsy-calibrated two-dimensional breath-hold Cartesian gradient echo (2D BH cGRE) R2*-MRI. Some MRI vendors offer corresponding three-dimensional (3D) GRE techniques (GE: IDEAL-IQ, Philips: mDixon, Siemens: LiverLab) with inline post-processing, enabling comprehensive liver coverage and generating R2* maps in a single breath-hold. However, breath-holding is impractical for pediatric or respiratory-compromised patients, and 3D free-breathing radial GRE (3D FB rGRE) has emerged as a viable alternative. Mean liver R2* values are estimated by manually drawing region-of-interest (ROI) either on a small homogenous area of liver without vessels or the whole liver and excluding vessels using postprocessing. Although the whole liver ROI reduces the inter-reviewer variability, both methods still require a trained expert and are time consuming. Our lab focuses on automating the segmentation of whole liver parenchyma on different BH and FB MRI sequences using a generalized convolutional neural network (CNN) for improving the workflow and accuracy of R2*-based HIC reporting for clinical diagnosis and treatment of iron overload.
Deep Learning for Sarcopenia Assessment
Sarcopenia is the decrease of muscle mass and has been associated in clinical outcomes in cirrhotic patients. Sarcopenia can be measured with dual-energy X-ray absorptiometry, computerized tomography (CT), and magnetic resonance imaging (MRI). Although CT is most widely used for sarcopenia, MRI is a more advantageous modality due to its ability to also determine lipid content with the Dixon method, also used for liver steatosis evaluation. CT studies measure sarcopenia with skeletal muscle index (SMI), the proportion of transverse cross-sectional area and height squared. In collaboration with Northwell Health, we are developing deep learning models to automatically segment paraspinal muscle to decrease the burden of manual segmentation, thereby increasing the potential of SMI being used clinically and in research.
NMR Metabolomics
Our lab quantifies metabolites in biological tissue samples using NMR spectroscopy to identify novel disease biomarkers and assess the effects of dietary interventions on the metabolome for improved diagnosis and prevention of metabolic diseases. Compared to other spectroscopic techniques, the advantages of NMR are it is highly reproducible, provides direct quantitative information and there is no destruction of sample. Further, an important benefit is that the in vitro metabolites detected using NMR, if abundant in the tissue of interest, can be followed noninvasively using high-field clinical MR scanners via in vivo magnetic resonance spectroscopy (MRS) for noninvasive diagnosis and monitoring of diseases and diet interventions. Our lab (a) develops protocols for preparing and extracting aqueous and lipid fractions from biological tissue samples using optimum solvent mixtures, (b) optimizes NMR scan parameters and temperature conditions for shifting and suppressing water peak and acquiring highly resolvable aqueous and lipid spectra, (c) investigates new spectral processing techniques to increase automation, reproducibility and accuracy in quantifying metabolites, and (d) finally validates the techniques in aqueous and lipid standards and in serum and tissue samples from animals fed with different diets.
Standards and Complex Mixtures
Obtaining measurements using analytical standards is important for validation before acquiring NMR spectra of biological samples. NMR samples of individual metabolite standards are prepared to determine each metabolite’s NMR fingerprint, and then complex lipid and aqueous mixtures are prepared with biologically relevant concentrations. This allows us to validate our procedures for metabolomics research and to experiment with new pulse sequences.
Tissue Samples
This is a collaborative project with the College of Health Sciences involving the NMR analysis of tissue samples from various dietary intervention studies. Lipids and aqueous metabolites are extracted from tissues such as liver, muscle, or serum and their concentrations are measured by NMR spectroscopy. This quantitative metabolomic analyses will provide complementary phenotypic information to other ‘-omics’ field for assessing the effects of nutrition on the metabolome for improved diagnosis and prevention of metabolic diseases.
NMR Metabolomics Workflow in Tissue Samples
Neural Network-based NMR Metabolite Profiling
Nuclear magnetic resonance (NMR) spectroscopy is a powerful tool for quantitative metabolomics; however, quantification of metabolites from NMR data is often a slow and tedious process requiring user input and expertise. This research highlights the potential of a neural network approach for improving speed and automation in NMR lipid profiling. A multi-layered perceptron was trained using a synthetically generated dataset and is able to quantify lipids in 5000 spectra in under half a second. The network accurately quantifies lipid species in experimentally acquired 1H-NMR spectra of lipid reference standards and achieved comparable statistical results to a more conventional quantification approach in analyzing lipophilic hepatic extracts from a dietary murine study. Code and raw data related to this study can be accessed at: https://github.com/tpirneni/mlp_nmr_lipids
Neural Network Workflow for Metabolite Profiling
Collaborative Projects
Automated Segmentation of Brain Volumes and Association with Auditory Function
This is a collaborative project with Dr. Bidelman, Auditory Cognitive Neuroscience Lab for investigating if there are any significant associations between brain structure and auditory function and determine any potential anatomical biomarkers for auditory perception and cognition. We have developed a pipeline to register and automatically segment brain volumes on MRI images including white matter and gray matter volumes for certain region-of-interests (ROIs) in the cortex. We will correlate these volumes to electroencephalography (EEG) data and other auditory and cognitive measures to investigate for potential neuroanatomical correlates.
Segmentation of Cortical Regions in Brain
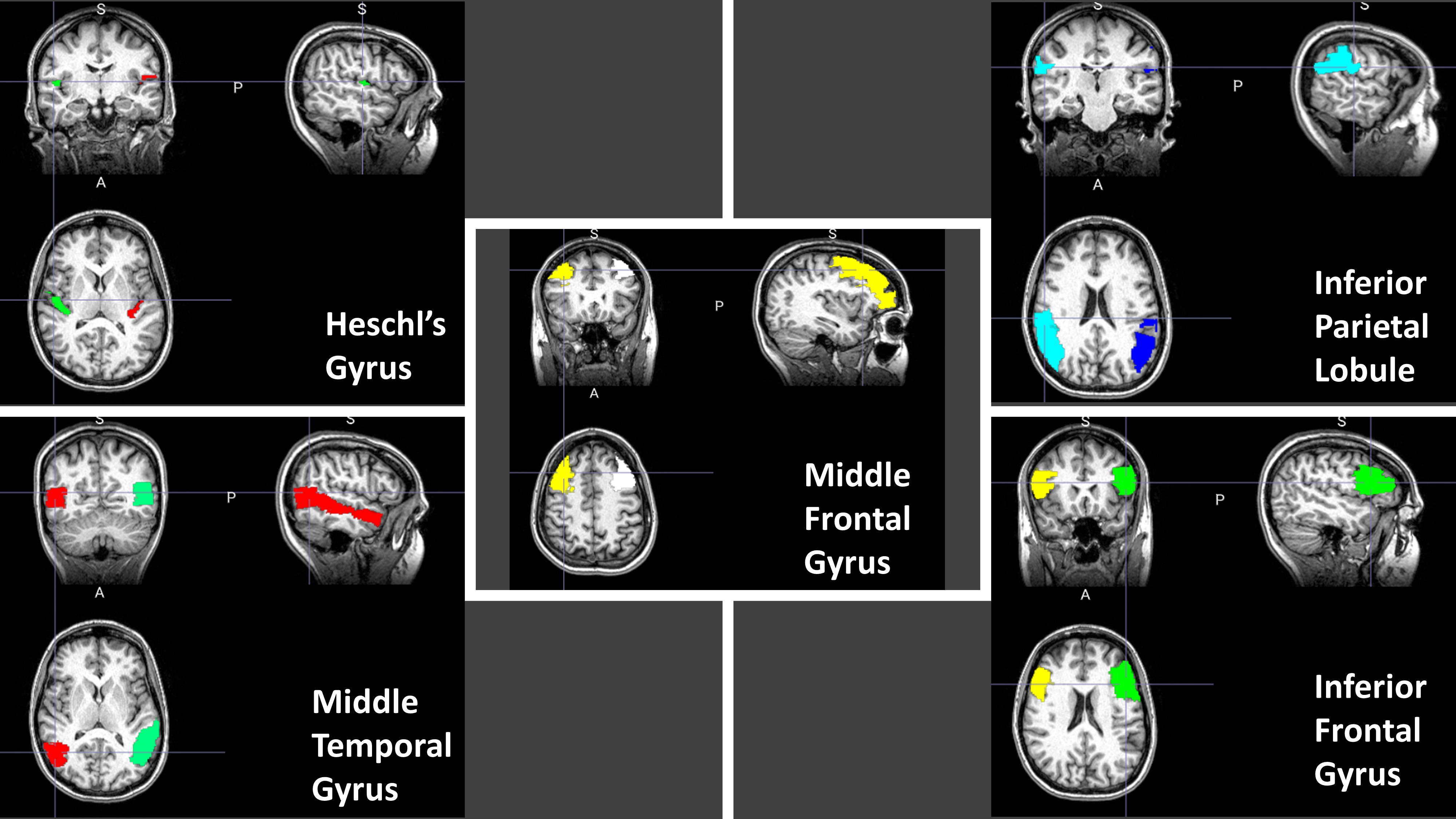